SnakeCLEF2021 - Snake Species Identification Challenge
Classify images of snake species from around the world
Starter Kit : https://github.com/AIcrowd/snake-species-identification-challenge-starter-kit
Description
Snakebite is the most deadly neglected tropical disease (NTD), being responsible for a dramatic humanitarian crisis in global health
Snakebite causes over 100,000 human deaths and 400,000 victims of disability and disfigurement globally every year. It affects poor and rural communities in developing countries, which host the highest venomous snake diversity and the highest-burden of snakebite due to limited medical expertise and access to antivenoms
Antivenoms can be life‐saving when correctly administered but this often depends on the correct taxonomic identification (i.e. family, genus, species) of the biting snake. Snake identification is also important for improving our understanding of snake diversity and distribution in a given area (i.e. snake ecology) as well as the impact of different snakes on human populations (e.g. snakebite epidemiology). But snake identification is challenging due to:
- their high diversity
- the incomplete or misleading information provided by snakebite victims
- the lack of knowledge or resources in herpetology that healthcare professionals have
In this challenge, we want to explore how Machine Learning can help with snake identification, in order to potentially reduce erroneous and delayed healthcare actions and improve snakebite eco-epidemiological data.
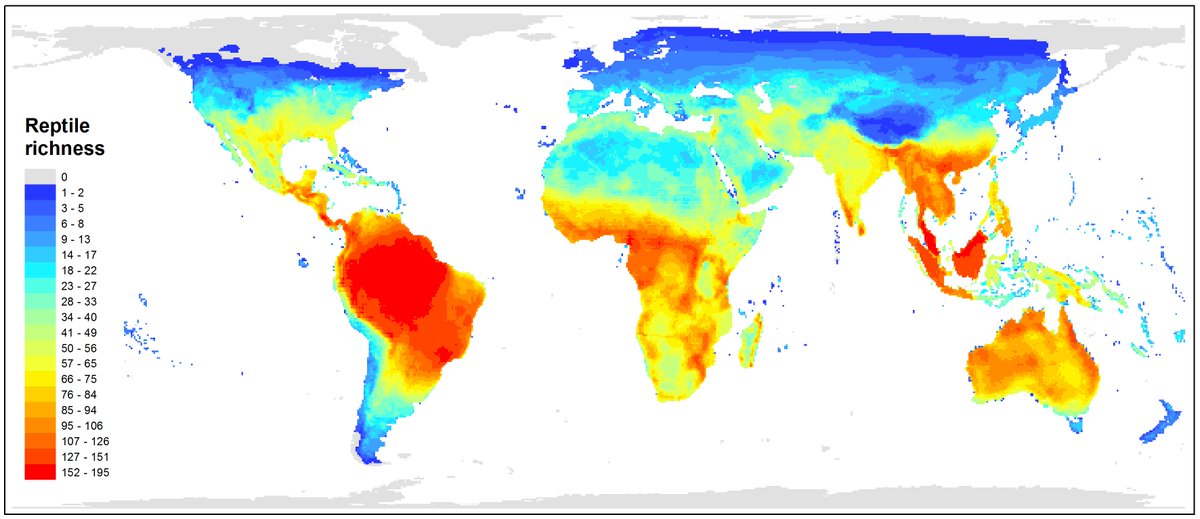
Species richness of reptiles worldwide
Tasks
In this challenge, you will be provided with a dataset of RGB images of snakes, and their corresponding species (class) and geographic location (continent, country). The goal is to create a system that is capable of automatically categorizing snakes on the species level.
- Some species have patterns that vary depending on their age
- Some species have patterns that vary depending on their location
- Two species might look very similar, with one being venomous and the other not
![]() |
![]() |
Metric 1
The primary metric for this year is a macro averaged F1 across countries.
- First, we calculate the F1 for each species (F1-S).
- Second, we calculate F1 for each countries using relevant F1-S scores for each country. F1-C
- Third, we calculate a mean based on F1-C Macro.
(The list with the species to country relations can be downloaded in a Resources tab.)
MEtric 2
The second metric for this year will be a macro averaged F1.
Datasets
Snakes are extremely diverse, and snake biologists continue to document & describe snake diversity, with an average of 33 new species described per year since the year 2000. Although most people probably think of snakes as a single “kind” of animal, humans are as evolutionarily close to whales as pythons are to rattlesnakes, so snakes in fact are very diverse! Taxonomically speaking, snakes are classified into 24 families, containing 529 genera and 3,789 species.
Distribution and Ordering
For this challenge, we prepared a large dataset with 414,424 photographs belonging to 772 snake species and taken in 188 countries. The majority of the data were gathered from online biodiversity platforms (i.e.,iNaturalist, HerpMapper) and were further extended with noisy data scraped from Flickr containing wrong labels and out-of-scope (non-snake) images. Furthermore, we have assembled a total of 28,418 correctly-identified images from private collections and museums used for testing. The final dataset has a heavy long-tailed class distribution, where the most frequent species (Thamnophis sirtalis) is represented by 22,163 images and the least frequent by just 10 (Achalinus formosanus). Such a distribution with small inter-class variance, high intra-class variance, and a high number of species (classes) creates a challenging task even for current state-of-the-art classification approaches.
![]() |
![]() |
Training and validation split
To allow participants to validate their intermediate results easily, we have split the full dataset into a training subset with 347,406 images, and validation sub-set with 38,601 images. Both subsets have the same class distribution, while the minimum number of validation images per class is one.
Subset
# of images
% of data
min. # of images/class
Training
347,405
83.83%
9
Validation
38,601
9.31%
1
Testing
28,418
6.86%
1
Total
414,424
100%
11
Testing Set
The final testing set remains undisclosed as it is composed of private images from individuals and natural history museums who have not put those images online in any form. A brief description of this final testing set is as follows: twice as big as the validation set, contains all 772 classes, similar class distribution, and observations from almost all the countries presented in training and validation sets.
Evaluation
Every participant has to submit their whole solution into the GitLab-based evaluation system, which performs evaluation over the undisclosed testing set. Since testing data is secret, each participating team can submit up to 1 submission per day. The primary evaluation metric for this challenge is a macro-averaged F1 score calculated across countries. The secondary metric will be a common multi-class macro-averaged F1 score.
Prizes
- USD 5K as part of Microsoft's AI for earth program Prize Money
- 3 authorships for top scoring teams
!! TEAMS that submit their work described in the working notes paper are eligible to receive the 5k USD price from Microsoft. !!
Timeline
- February 2021: Training and validation data release.
- 16 May 2021 - 9 pm CET: Deadline for submission of runs by participants.
- 17 May 2021: The release of processed results by the task organizers.
- 28 May 2021: Deadline for submission of working note papers by participants [CEUR-WS proceedings].
- 11 June 2021: Notification of acceptance of working note papers [CEUR-WS proceedings].
- 2 July 2021: Deadline for camera ready working note papers by participants and organizers.
- 21-24 Sept 2021: CLEF 2021 Bucharest, Romania
Contact us
If you have any problems or comments, please contact us via the discussion forum or via email:
Lukas Picek: lukaspicek@gmail.com
Andrew Durso: amdurso@gmail.com
Rafael Ruiz de Castaneda: rafael.ruizdecastaneda@unige.ch
Isabelle Bolon: isabelle.bolon@unige.ch
Participants

















































