Success story
SBB: Improving Train Timetables

SBB, the Swiss Federal Railways, manages one of the most densely-used mixed-traffic railway networks in the world. Every day, SBB transports over 1.2 million people and carries 210’000 ton-kilometers of freight on more than 10’000 trains, all while achieving world-leading punctuality metrics.
While average utilisation of the SBB infrastructure (measured in average number of trains per kilometer of track) is already second-to-none, traffic is expected to increase even further. There are also financial incentives to use the available infrastructure to its maximum. However, before trains can run, they must first be accepted into the timetable.
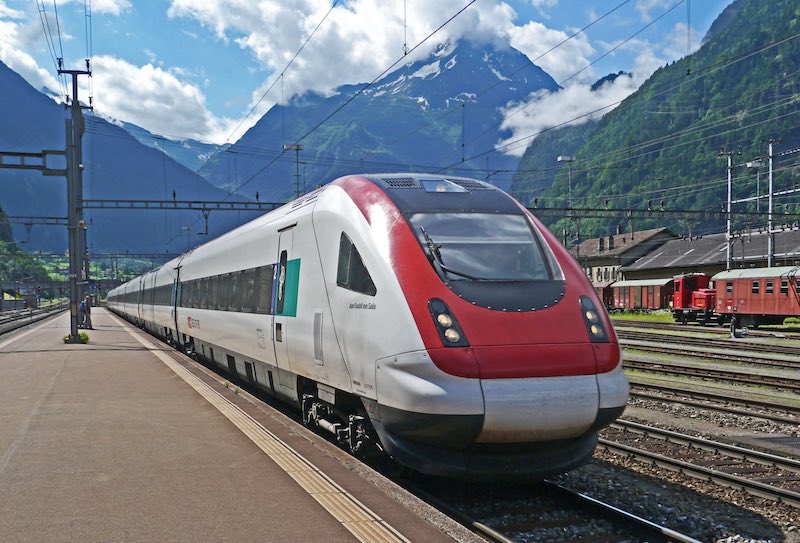
Generating a railway timetable is known to be an NP-hard problem. While scheduling a few trains is easy, complications quickly explode with an increasing number of trains. SBB was interested in using a crowdsourcing challenge to solicit ingenious ways to tackle the timetable generation/optimization problem.
Julian Jordi and Erik Nygren, who coordinated the project at SBB, saw the benefits of crowdsourcing as a great way to address this problem because “it encourages a broad community with a very diverse background to reflect on your problem and come up with wide variety of solution approaches”.
The top solutions all used different technologies and algorithms, and they all performed very well.
We worked with Erik’s team to develop the challenge, where SBB provided participants with a set of sample problem instances consisting of a list of trains to be scheduled, their commercial requirements to be respected, and a set of routes they can take through the network. The task was to come up with a timetable for these problem instances.
“Initially, we were worried that the problem description was a bit too complicated”, Julian reflected after the challenge. “After all, participants had only three months to solve the problems; and before you can solve them, you first need to understand what exactly the question is. But in the end, one of the biggest surprises was how easily many participants absorbed all the business logic encoded in the problems.”
Crowdsourcing far exceeded our expectations - you not only get new solutions, but also a deeper insight to the problem you are trying to solve.
Immediately after challenge launch, the team at SBB realized that the crowdsourcing approach worked much better than they had anticipated. “48h after the start of the challenge, someone had submitted feasible solutions to all 9 problem instances we provided”, recalls Julian Jordi who coordinated the technical aspects of the project at SBB. “This demonstrated that the participants could get an understanding of our problem and data models quite quickly. This was really unexpected.”
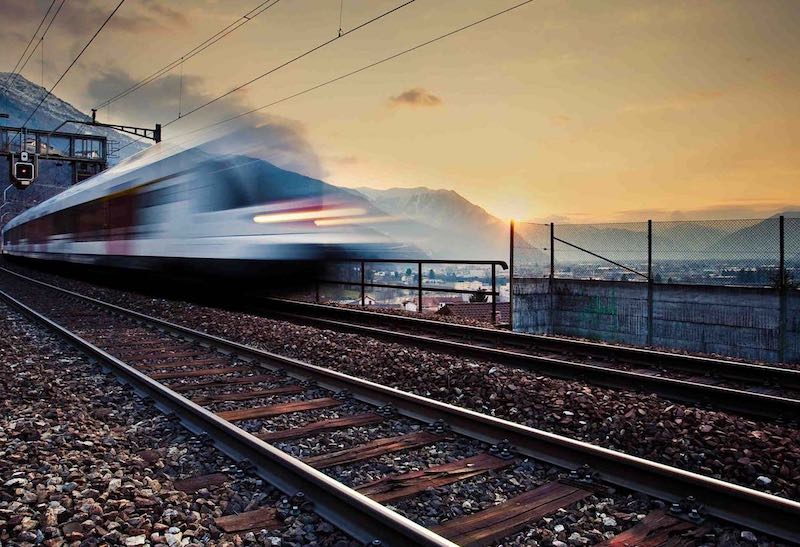
The challenge ended with a lot of great solutions. “The top solutions all used different technologies and algorithms, and they all performed very well. And the ideas behind the algorithms have, to the best of our knowledge, not been tried yet in the academic literature”, said Julian while reflecting on the future of crowdsourcing. And Erik added, “Crowdsourcing far exceeded our expectations - you not only get new solutions, but also a deeper insight to the problem you are trying to solve.”
Would they do it again? “Emphatically yes!”, says Erik, who is now coordinating a new multi-agent reinforcement learning challenge by SBB on the AIcrowd platform, the Flatland Challenge, with a bigger team and more resources. The reasons for working with AIcrowd on the new challenge are the same as they were for the first challenge: “The strong connection to academia, the great track record, and the transparent and down-to-earth communication.”
Interested in hosting a challenge?
Then head over to our organize a challenge page to get the ball rolling.